Using Zarr for images – The OME-ZARR standard
We can use the Zarr file format for storing image files such as for any other NumPy array. In this post we additionally explore the NGFF (next-generation file format) OME-ZARR standard for storing images with Zarr.
Let’s first prepare an example image by loading the cells3d image from the scikit-image
package:
import numpy as np
from skimage import data, exposure
import plotly
import plotly.express as px
import plotly.io as pio
pio.renderers.default = "browser"
# load 4D image (3D + 2 channels) from the skimage samples:
array_3D = data.cells3d()
# select the nuclei channel and refine the image depth:
array_3D = array_3D[22:54,1,:,:]
image_shape = array_3D.shape
print(image_shape)
# define a function for rescaling the intensity of each
# layer to enhance the visibility:
def enhance(image):
vmin, vmax = np.percentile(image, q=(0.5, 99.5))
image = exposure.rescale_intensity(image, in_range=(vmin, vmax),
out_range=np.float32 )
return image
# plot with plotly into the default browser:
fig = px.imshow(enhance(array_3D), animation_frame=0,
binary_string=True, binary_format='jpg')
plotly.io.show(fig)
Storing images using default Zarr methods
We can store that image like any other NumPy array as a Zarr file to the disk using default Zarr i/o-syntax:
import zarr
# write the image as a Zarr array to disk:
chunks = (1, image_shape[1], image_shape[2])
zarr_out_3D = zarr.open('zarr_3D_image.zarr', mode='w',
shape=array_3D.shape,
chunks=chunks,
dtype=array_3D.dtype)
zarr_out_3D[:] = array_3D
# reopen/read the Zarr array:
zarr_in_3D = zarr.open('zarr_3D_image.zarr')
fig = px.imshow(enhance(zarr_in_3D[:]), animation_frame=0,
binary_string=True, binary_format='jpg')
plotly.io.show(fig)
The generated plot is identical to the one shown above. For the remainder of this post I will not show that plot and refer to the one above.
The OME-NGFF/OME-ZARR standard
In 2020/2021, the Open Microscopy Environmentꜛ (OME) has proposedꜛ the next-generation file format (NGFF) specificationsꜛ (GitHubꜛ) for storing multi-resolution bioimaging data in the cloud. The OME defines this OME-NGFF called standard based on the Zarr file format, which provides the necessary support for storing and accessing arrays from distributed cloud storages. I will therefore refer to that standard as OME-ZARR for the remainder of this post.
Even though being proposed for the usage of images in the cloud, we can use it as a general standard for storing images as Zarr arrays (like the OME-TIFFꜛ specifications for storing TIFF files). The advantages of using such a standard are:
- standardization of the metadata (regarding both format and name definition, according the OME-XML structured annotations specificationsꜛ),
- standardization of the structure within the Zarr file (i.e., how the image is stored within the Zarr fileꜛ), and
- standardization of the Zarr-store format (Zarr storage specificationsꜛ).
This standardization enables developers to easily provide an API, that works for all OME-ZARR files in a unified manner. We can write image analysis pipelines and don’t have to care, how the hierarchy within a Zarr files might be organized – we can expect it to always be arranged and accessible in the same way. The standardization of the metadata also sets a clear frame for how to store and name image attributes such as the microscope metadata, image resolution or the channel specifications.
OME-ZARR with Python
In Python, the OME-ZARR standard is provided by the ome-zarr-py
ꜛ package. Let’s take a look, how to store and read our example image from above accordingly:
from ome_zarr.io import parse_url
from ome_zarr.writer import write_image
from ome_zarr.reader import Reader
store = parse_url("zarr_3D_image.ome.zarr", mode="w").store
root = zarr.group(store=store, overwrite=True)
write_image(image=array_3D, group=root, axes="zyx",
storage_options=dict(chunks=chunks))
The parse_url(...).store
ꜛ function creates a Zarr directory store in a specific format ("FormatV04"
) and with a specific dimension separator ("/"
). This is equivalent to:
store = zarr.storage.FSStore("zarr_3D_image.ome.zarr", mode="w",
format="FormatV04",
dimension_separator="/")
root = zarr.group(store=store, overwrite=True)
write_image(image=array_3D, group=root, axes="zyx",
storage_options=dict(chunks=chunks, overwrite=True))
The write_image()
ꜛ function saves the image to the created group (root
) into the Zarr file by creating a pyramid of resolution levels (the default is five levels), where each image layer is down sampled by a factor of 2 with each level. This is why the stored image array actually consists of five sub-arrays/-folders within the Zarr file:
print(root.info)
print(root.tree())
Name : /
Type : zarr.hierarchy.Group
Read-only : False
Store type : zarr.storage.FSStore
No. members : 5
No. arrays : 5
No. groups : 0
Arrays : 0, 1, 2, 3, 4
/
├── 0 (32, 256, 256) uint16
├── 1 (32, 128, 128) uint16
├── 2 (32, 64, 64) uint16
├── 3 (32, 32, 32) uint16
└── 4 (32, 16, 16) uint16
With the knowledge about the internal file structure, we can read the OME-ZARR file by using default Zarr i/o-syntax:
zarr_in_3D = zarr.open("zarr_3D_image.ome.zarr")
fig = px.imshow(enhance(zarr_in_3D["0"][:]), animation_frame=0,
binary_string=True, binary_format='jpg')
plotly.io.show(fig)
The ome-zarr-py
packages also provides its own reader function, Reader()
ꜛ:
# read the OME-ZARR file with the ome_zarr io-method:
reader = Reader(parse_url("zarr_3D_image.ome.zarr"))
# nodes may include images, labels etc.:
nodes = list(reader())
# first node will be the image pixel data at full resolution:
image_node = nodes[0]
zarr_in_3D = image_node.data
fig = px.imshow(zarr_in_3D[0][:], animation_frame=0,binary_string=True, binary_format='jpg')
plotly.io.show(fig)
Adding metadata
We can add OME-XMLꜛ-like metadata to the stored image array by assigning Zarr attributes. We follow the example from the ome-zarr-py
documentation websiteꜛ and add some omero-styleꜛ rendering settings:
root.attrs["omero"] = {
"channels": [{
"color": "00FFFF",
"window": {"start": 0, "end": 20},
"label": "nuclei",
"active": True,
}]
}
Storing multiple images into one OME-ZARR file
We can also add more than one image to an OME-ZARR file by adding groups to the Zarr store:
store = zarr.storage.FSStore("zarr_3D_image_groups.ome.zarr",
mode="w",
format="FormatV04",
dimension_separator="/")
root = zarr.group(store=store, overwrite=True)
root_sub_1 = root.create_group("sub_array_1", overwrite=True)
root_sub_2 = root.create_group("sub_array_2", overwrite=True)
root_sub_3 = root.create_group("sub_array_2/sub_sub_array_1", overwrite=True)
write_image(image=array_3D, group=root, axes="zyx",
storage_options=dict(chunks=chunks, overwrite=True))
write_image(image=array_3D, group=root_sub_1, axes="zyx",
storage_options=dict(chunks=chunks, overwrite=True))
write_image(image=array_3D, group=root_sub_2, axes="zyx",
storage_options=dict(chunks=chunks, overwrite=True))
write_image(image=array_3D, group=root_sub_3, axes="zyx",
storage_options=dict(chunks=chunks, overwrite=True))
print(root.tree())
/
├── 0 (32, 256, 256) uint16
├── 1 (32, 128, 128) uint16
├── 2 (32, 64, 64) uint16
├── 3 (32, 32, 32) uint16
├── 4 (32, 16, 16) uint16
├── sub_array_1
│ ├── 0 (32, 256, 256) uint16
│ ├── 1 (32, 128, 128) uint16
│ ├── 2 (32, 64, 64) uint16
│ ├── 3 (32, 32, 32) uint16
│ └── 4 (32, 16, 16) uint16
└── sub_array_2
├── 0 (32, 256, 256) uint16
├── 1 (32, 128, 128) uint16
├── 2 (32, 64, 64) uint16
├── 3 (32, 32, 32) uint16
├── 4 (32, 16, 16) uint16
└── sub_sub_array_1
├── 0 (32, 256, 256) uint16
├── 1 (32, 128, 128) uint16
├── 2 (32, 64, 64) uint16
├── 3 (32, 32, 32) uint16
└── 4 (32, 16, 16) uint16
OME-ZARR arrays stored into groups can be accessed like default OME-ZARR arrays:
zarr_in_3D = zarr.open("zarr_3D_image_groups.ome.zarr")
fig = px.imshow(enhance(zarr_in_3D["sub_array_1"]["0"][:]), animation_frame=0,
binary_string=True, binary_format='jpg')
plotly.io.show(fig)
Adding labels
It is also possible to add labels directly to the OME-ZARR store. Let’s calculate some labels for our example image:
from skimage import segmentation as seg
from scipy import ndimage as ndi
from skimage.feature import peak_local_max
from skimage import filters
from scipy import ndimage
import matplotlib.pyplot as plt
def plot_projection(array3D, title="dummy 3D stack", projection_method="mean", axis=2):
"""
Plot function: plots a 2D average intensity z-projection of an input 3D array.
"""
fig = plt.figure(2, figsize=(5, 5))
plt.clf()
if projection_method =="mean":
plt.imshow(array3D.mean(axis=axis))
plt.title(title + "\naverage intensity z-projection", fontweight="bold")
elif projection_method=="max":
plt.imshow(array3D.max(axis=axis))
plt.title(title+"\nmaximum intensity z-projection", fontweight="bold")
plt.xlabel("x-axis", fontweight="bold")
plt.ylabel("y-axis", fontweight="bold")
plt.colorbar()
plt.tight_layout()
plt.show()
plt.savefig(title+" projected.png", dpi=120)
# pre-filter the image stack:
array_3D_filtered = ndimage.median_filter(array_3D, size=7)
array_3D_filtered = filters.gaussian(array_3D_filtered, sigma=2)
# threshold:
threshold = filters.threshold_otsu(array_3D_filtered)
array_3D_threshold = array_3D_filtered > threshold
# segment array_3D_threshold via the watershed method:
distance = ndi.distance_transform_edt(array_3D_threshold.astype("bool"))
max_coords = peak_local_max(distance, min_distance=10,labels=array_3D_threshold.astype("bool"))
local_maxima = np.zeros_like(array_3D_threshold, dtype=bool)
local_maxima[tuple(max_coords.T)] = True
markers = ndi.label(local_maxima)[0]
labels = seg.watershed(-distance, markers, mask=array_3D_threshold.astype("bool"))
# some control plots:
plot_projection(labels, title="dummy 3D stack labels", projection_method="max", axis=0)
plot_projection(enhance(array_3D), title="dummy 3D stack", projection_method="mean", axis=0)
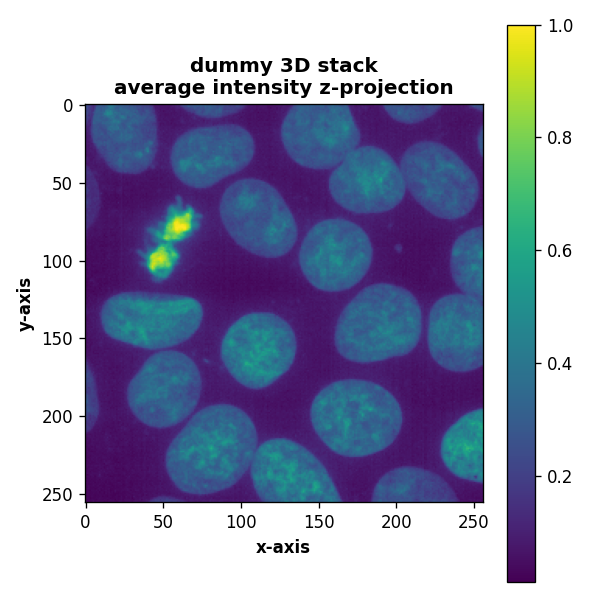
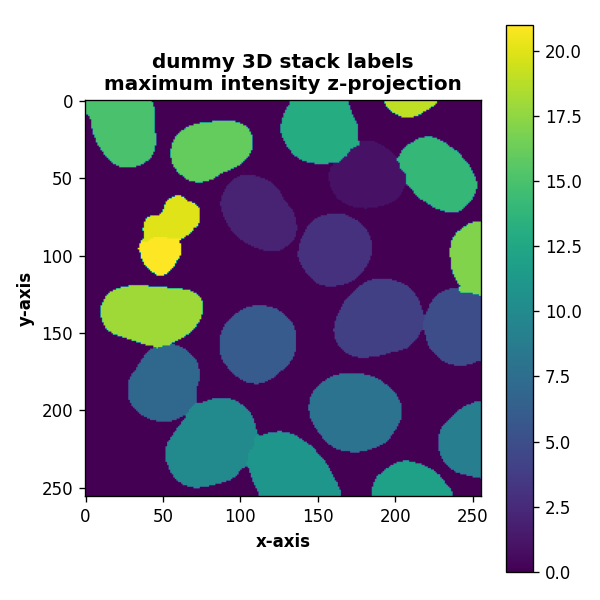
Now we write the labels to the OME-ZARR directory:
# create the OME-ZARR store:
store = parse_url("zarr_3D_image.ome.zarr", mode="w").store
root = zarr.group(store=store, overwrite=True)
write_image(image=array_3D, group=root, axes="zyx",
storage_options=dict(chunks=chunks))
# write the labels to "/labels":
labels_grp = root.create_group("labels", overwrite=True)
label_name = "watershed"
labels_grp.attrs["labels"] = [label_name]
label_grp = labels_grp.create_group(label_name)
# the 'image-label' attribute is required to be recognized as label:
label_grp.attrs["image-label"] = { }
write_image(labels, label_grp, axes="zyx")
# control plot:
zarr_in_3D = zarr.open("zarr_3D_image.ome.zarr")
plot_projection(zarr_in_3D["labels/watershed"]["0"][:],
title="dummy 3D stack read labels",
projection_method="max", axis=0)
OME-ZARR in Napari
Napariꜛ is able to read OME-ZARR files via the napari-ome-zarr
ꜛ plugin:
Image taken from https://www.napari-hub.org/plugins/napari-ome-zarr.
With that plugin, we can simply drag and drop our example OME-ZARR folder “zarr_3D_image.ome.zarr” into the Napari main window, and the image and the associated labels are read accordingly:
We can also pass the image to Napari via Python by opening the OME-ZARR file (or any other Zarr file) and handing over the desired Zarr array to Napari:
zarr_in_3D = zarr.open("zarr_3D_image.ome.zarr")
viewer = napari.view_image(enhance(zarr_in_3D["0"][:]))
labels_layer = viewer.add_labels(zarr_in_3D["labels/watershed"]["0"][:],
name='watershed')
By now, Napari is not the only image viewer that provides support for OME-ZARR files. There is for example Vizarrꜛ, and in my next post I will show, how to read OME-ZARR files in Fiji.
Accessing OME-ZARR images stored in the cloud
Finally, let’s see how we can access OME-ZARR images that are stored in the cloud:
path = "https://uk1s3.embassy.ebi.ac.uk/idr/zarr/v0.1/6001246.zarr/"
store = parse_url(path, mode="r").store
reader = Reader(parse_url(path))
nodes = list(reader())
image_node = nodes[0]
read_data = image_node.data
viewer = napari.view_image(read_data[0], channel_axis=0)
You can find more publicly available OME-ZARR samples hereꜛ and hereꜛ.
The Python code used in this post is also available in this GitHub repositoryꜛ.
Comments
Commenting on this post is currently disabled.
Comments on this website are based on a Mastodon-powered comment system. Learn more about it here.